Accuracy of AI Modals with Image Annotation Company Image Annotation Services:
Image annotation refers to the act of strategically identifying an image. It may involve human labor as well as computer assistance. It is an important stage in developing computer vision models that can be used for object identification, classification, segmentation, or image segmentation. You can annotate every set of pixels for an image, or you might label the entire image with one label.
Image classification, bounding boxes, critical point annotations, LiDAR and semantic segmentation are some image annotation services that can tailor to a client’s needs. There are many deep-learning applications for picture annotation. These include autonomous technology & transport, medical AI and finance, government, GIS, and other sectors.
Image annotation services can accurately capture all sizes and types of photos to make them easily recognizable for computers or machine vision. We use the most advanced tools and methods to provide training data for machine learning in many subfields. It is done by annotating photos taken from different fields.
1. Bounding boxes:
This is the most common type of image annotation in computer vision. Rectangular box annotation is a technique that computer-vision professionals use to represent items and train data. It allows algorithms with annotated photographs to identify and locate objects during machine learning. Bounding boxes are simple, and this makes them useful for many purposes.
2. Polygon annotation:
Expert annotators mark each vertex of the target object with points. Polygon annotation allows precise annotation of each edge of an item, regardless of its shape. It allows models of artificial intelligence (and computer vision) to recognize and respond to objects. It is a beneficial method in computer vision because it allows annotators to recognize irregular shapes, and computers can recognize them.
3. Semantic segmentation:
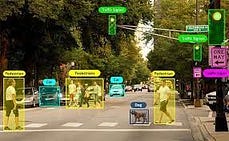
The team splits images into individual pieces and annotates each section. Computer vision specialists can identify desirable objects in photos at the individual pixel level. With the aid of skilled semantic segmentation, It can organize data in many ways to create AI models for various uses.
4. LiDAR Note:
Teams use 360-degree visibility photos taken by multi-sensor camera systems to identify ground truth data for computer vision models.
5. Image classification:
Because of unique multi-level taxonomies, such as land use, crops, and residential property attributes, among others, annotators categorize photos or objects inside images. AI and ML algorithms use expert image classification to transform image data into image insights. 6.3D Cuboid annotation: Cuboids allow to create training datasets to teach machine learning models how to recognize the depth of objects. Expert data labeling produces the best-in-class training datasets to help computer vision models identify obstacles and object dimensions. These dots will be connected to a line with anchor points to provide a 3D depiction. These anchor points are typically found close to the edges of the object.
We offer image annotation company for machine learning and AI-oriented companies that need such data sets with the highest accuracy. Annotated images help computers and machines recognize objects through their dimensions and outlined boxes. This data can be used for future reference and to identify similar objects.
Our professionals can provide the best possible results for all your picture annotation needs. Our image annotation outsourcing services are affordable and of the highest quality.
Key mark, a dedicated service that handles data annotation, can save computer vision developers’ time. It also ensures timely and accurate image annotation. Human employees must locate, outline and label individuals and items to annotate photos. An extensive annotation process is required for many photos.
Auto-labeling platforms make it easy to create training datasets quickly. This system reduces the manual labeling that people must do, which can save time and money. However, more reliance on automated annotation can be needed to improve the quality of image data.
Many AI-assisted image labeling options can speed up the process of annotating images. It can be as simple as auto-labeling items from thousands of photos or as complex as creating polygon outlines for objects to aid in semantic segmentation.
Image Annotation Benefits:
Image annotation enhances deep learning models and increases precision in diagnosis. Computer vision models can recognize images from CT scans, MRI scans, and X-ray scans. The repository contains accurately labeled data. It can use Image annotation to benefit the security and surveillance industry greatly. To stop significant crimes, train computer vision models to recognize faces in crowds and examine human behavior.
You can still track the intruder even if they try blending in with the group using 2D or 3-D bounding boxes. They may be taught to recognize demographics and count people. ADAS annotation is used in prisons, public safety, security, government, private sectors, military base camps, radar centers, and other critical infrastructure. It is also helpful in protecting commercial buildings, such as banks, ATMs and airports. It also reduces the time it takes to scan patients and makes them less likely to wait.
Self-driving cars are not a new concept. Due to increased client demand and investment, companies like Uber and Tesla are investing more in their autonomous taxi technology and computer vision-based self-driving cars. Automakers can create intelligent apps that allow them to connect autonomously and self-driving cars by using the ability to annotate photos. Government sectors are also increasing digitization to create centralized, seamless traffic control. It is also driving data labeling and image annotation projects in the global transport sector.
Comments
Post a Comment